DIGITAL TWINS APPLICATIONS
PREDICTIVE MAINTENANCE
Definitions, Pros, Our System ...
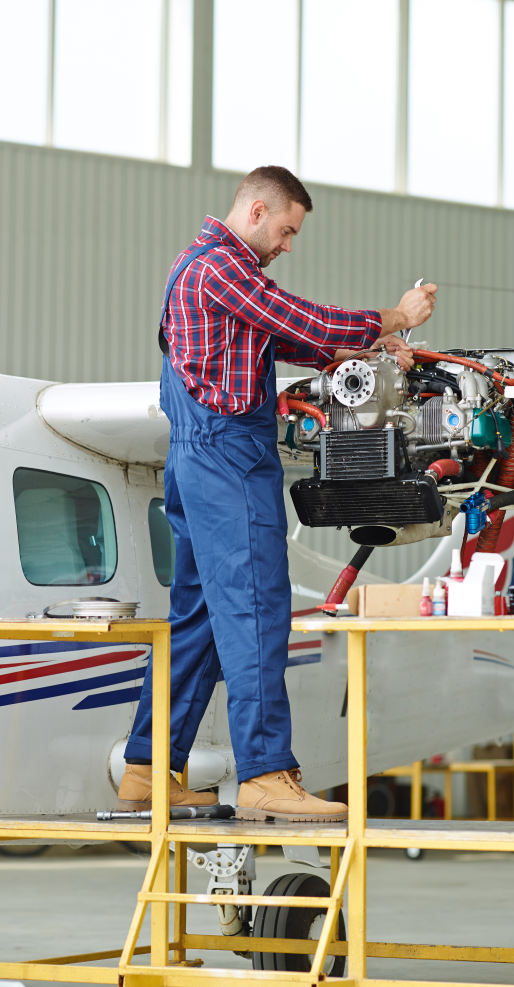
The Importance of Maintenance Actions
We always want our products available when We need to use them. An unexpected failure has different consequences in different systems. A failed component in an aircraft can avoid its departure, delaying flights, disturbing passengers and imposing fees to the airlines. The same happens with automobiles, where lower cost components not replaced on the right time can make higher cost components work harder, reducing their lifespan. In big production lines, a few hours interruption can cause millions of loss.
In all the examples above, maintenance actions are taken to maximize the availability of these systems.
This preventive maintenance actions are often specified during design phases defining regular intervals of inspection and components replacement, reducing the probability of corrective actions during unexpected interruptions.
But what happens when the operation of a system is not exactly the same of the typical operation considered in the design?
Predictive Maintenance
Now-a-days, using only the preventive maintenance strategy, when system operation differs from the typical operation considered in design, the likelihood of unexpected failures and the need for corrective maintenance actions increases substantially.
To solve this problem, predictive maintenance strategies have been developed, based on data from sensors coupled to systems, and mathematical models are developed to predict the probability of system failure, allowing the planning of interruptions before the failure occurs.
Because predictive maintenance is based on component usage information, you can anticipate or delay component replacement stops according to the collected data, extending the life of systems.
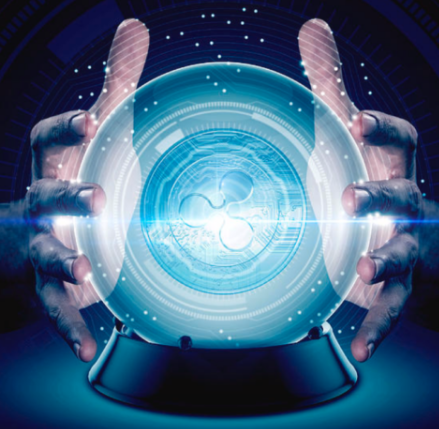
INTELLIGENT INSIGHTS FRAMEWORK
Our Framework applied to Predictive Maintenance
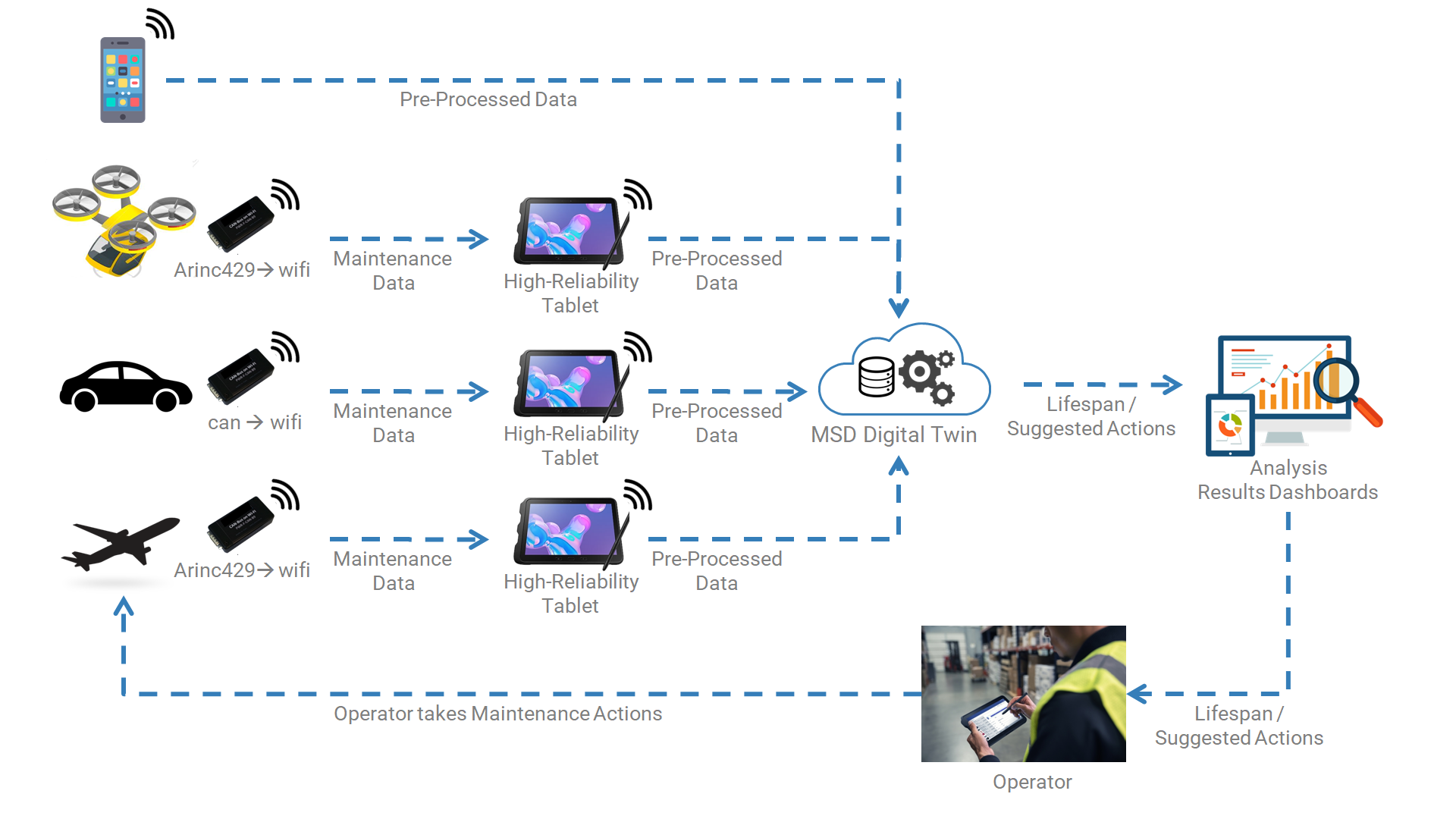
The figure above shows the integration of the Intelligent Insights framework into different systems, from smartphones and automobiles to aircraft and new drone systems used as air taxi.
Integration takes place from interface hardware that connects the maintenance system (usually using CAN protocol, ARINC-429, …) to a computer capable of connecting to our system. Generally, the maintenance environment requires portable devices and is subject to more demanding environmental conditions, so we suggest using highly reliable tablets.
The central part of the predictive maintenance system is the mathematical model capable of estimating the remaining life of the component of interest. This integrated system (the predictive maintenance-focused digital twins application) returns the remaining component life information to the operator, who can decide on the best time to replace components.
Different and Complex Systems... Which component do We choose to start?
Systems of this level of complexity have numerous completely different components, but in an “increasingly electric world,” everyone needs batteries. Batteries are not the most frequently replaced components, so we only remember them when they fail.
In aircraft, the “health” of the batteries is checked before each flight. If minimum conditions are not met, they must be replaced. This event is only detected at the moment the aircraft is energized, which may cause delays in flight preparation, and consequently fines the airlines.
In conventional cars, batteries are replaced in periodic reviews. However, it is so common for batteries to fail between overhauls that there are specialized “rescue” services that go to your vehicle just to replace the failed battery.
In hybrid or fully electric cars, batteries are responsible for a significant portion of the vehicle’s cost. Monitoring the remaining life can prevent replacement early, reducing maintenance costs, and giving owners more security. This type of monitoring also opens the possibility of feasibility studies considering lower cost batteries and shorter service life, since the replacement would take place on a planned basis. Or thinking about the near future where electric and hybrid cars will be more common, assessing the health of batteries in used vehicles will play a key role in defining resale value.
The same can be said of the new drone air taxi services, with the aggravation that battery failure in flight is a safety hazard for passengers.
Another system greatly affected by batteries is the smartphone. In this case the user will notice the battery gradually deteriorating, until he has to make a decision: replace only the battery or purchase a new device (with a new battery). Knowing when the user is most likely to make this replacement is extremely relevant information for smartphone manufacturers’ sales.
For all these reasons, we are applying digital twins to predictive maintenance. Our initial focus is on predicting battery life, but this is just the beginning. We are constantly evaluating other components and systems that may benefit from our technology.